Representation is crucial for being seen and accounted for by governments, businesses, and inter governmental agencies. It takes many forms, and being represented on a map is one of the most effective ways to accurately delineate voting districts, disaster management, infrastructure planning, medical supplies distribution, and navigation. Our project aimed to bridge the digital divide by developing an open-source toolkit for mapping buildings on high-resolution satellite imagery. Replicable AI for Microplanning (RAMP) is a powerful yet user-friendly machine-learning model that extracts buildings from satellite imagery. It can be used by anyone with a reasonably powerful laptop and high-resolution satellite/drone imagery of a specific area of interest.
RAMP builds on past efforts to map the “missing millions.” While Facebook, Google, and OpenStreetMap have released building footprint data, they have limitations. The documentation, codebase, and training data behind Facebook and Google’s releases have not been made available, and OpenStreetMap’s data is generated manually. RAMP aims to make every aspect of the project openly available, delivering baseline and fine-tuned models for footprint extraction to exceed 90% accuracy in building extraction across Bangladesh.
The RAMP project simplifies the use of machine learning (ML) for extracting building footprint data. It provides comprehensive documentation, Jupyter notebooks, and ample training data to create customized models for any location. RAMP simplifies preliminary mapping work by providing an open-source codebase
that is fully functional through Jupyter Notebooks. Organizations can focus on creating a small amount of training data to generate a new “fine-tuned” model optimized for their desired geography. We aim to shift data production to the regions where work is being done by creating a ‘building extraction toolkit.’ This open approach helps GIS analysts in LMICs, and individuals use our work to develop building footprint datasets without requiring expensive hardware or cloud computing. Once the localized training data is integrated, the GIS Analyst can respond quickly to their mapping needs.
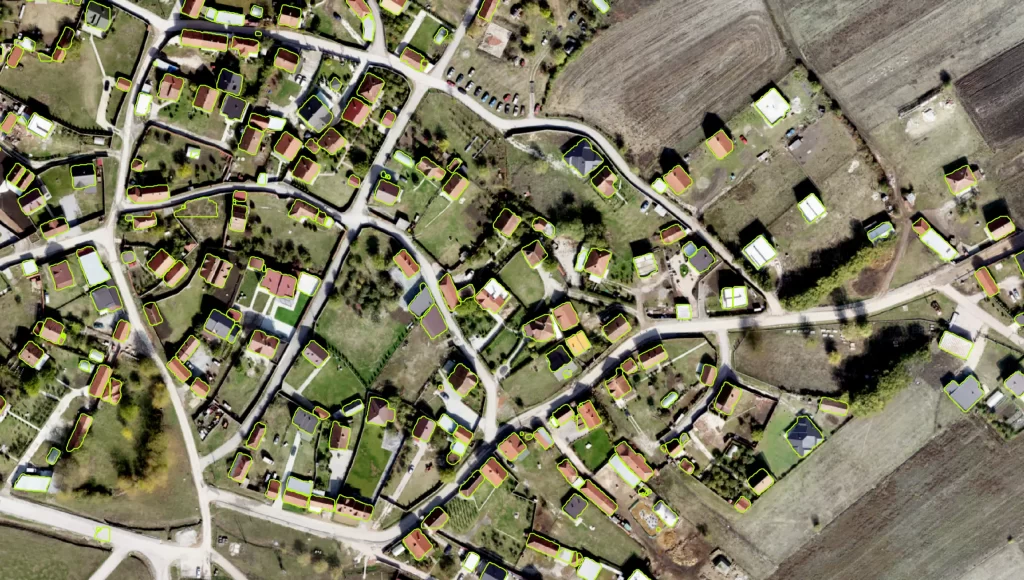
On-demand mapping in LMICs to support disaster planning/response and microplanning. In case of a flood in Laos, rapid response teams gather data to map the population distribution before the event. However, the data from sources like OSM and land records can be inaccurate and outdated. The Ramp project uses machine learning to extract building footprints from satellite imagery, reducing the time required for data creation. This time can be better utilized to plan a response with an accurate and up-to-date map.
Capacity enrichment at gov institutions, NGOs, and universities. Our project, RAMP, aims to create a ramp that benefits institutions and individuals immediately and acts as a launchpad for expanding the scope and outcomes of our first iteration. We will provide thorough documentation, training data, and code for global development and humanitarian communities to integrate into their map production workflows. This integration will spark innovative ideas on how our approach can serve program needs beyond our current vision.
Very interesting info!Perfect just what I was
looking for!Raise blog range
Can you be more specific about the content of your article? After reading it, I still have some doubts. Hope you can help me.